成果速递:硕士生姚啸宇发表最新研究成果
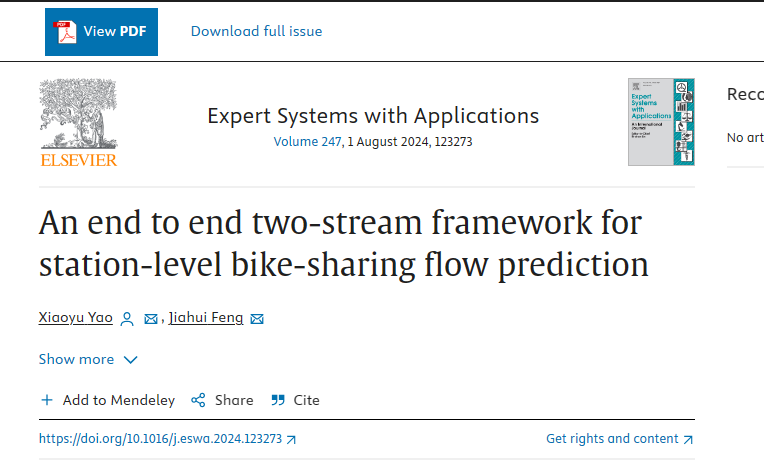
Title: An End to End Two-stream Framework for Station-level Bike-sharing Flow Prediction;
Authors: Xiaoyu Yao*, Jiahui Feng;
Journal: Expert Systems with Applications;
URL:https://doi.org/10.1016/j.eswa.2024.123273;
Abstract:
The growth of bike-sharing systems worldwide has led to an increased need for accurate bike-sharing flow prediction at the station level. However, previous studies mostly overlooked both substantial heterogeneity and strong correlations between the returned and rented bike flows among bike stations. This study proposes the Two-Stream Station-level Bike-sharing Flow Prediction (TS-SBFP) framework that incorporates single-stream feature extraction, cross-stream feature interaction, global information embedding, and two-stream feature fusion for station-level bike-sharing flow prediction. In this framework, inflow and outflow data are separated into two streams and input to the prediction model respectively. First, multiscale gated convolution is applied in both streams to extract abundant temporal correlations. Second, two-stream graph attention is applied to capture rich local spatial correlations between the inflows and outflows among bike stations. Furthermore, spatiotemporal fusion network is designed to effectively and efficiently fuse the two-stream features. Additionally, to identify the predictive contributions of bike stations, global station-aware attention is designed to acquire the importance of each bike station using the squeeze-and-excitation mechanism. Finally, experiments conducted on three real-world datasets demonstrate the effectiveness of the proposed approach. In addition, two-stream attention visualization and analysis verify that the proposed approach can adaptively learn spatiotemporal interaction patterns.Acknowledgements: This work was supported by the National Natural Science Foundation of China (NSFC: 72332007).